Hallucinations
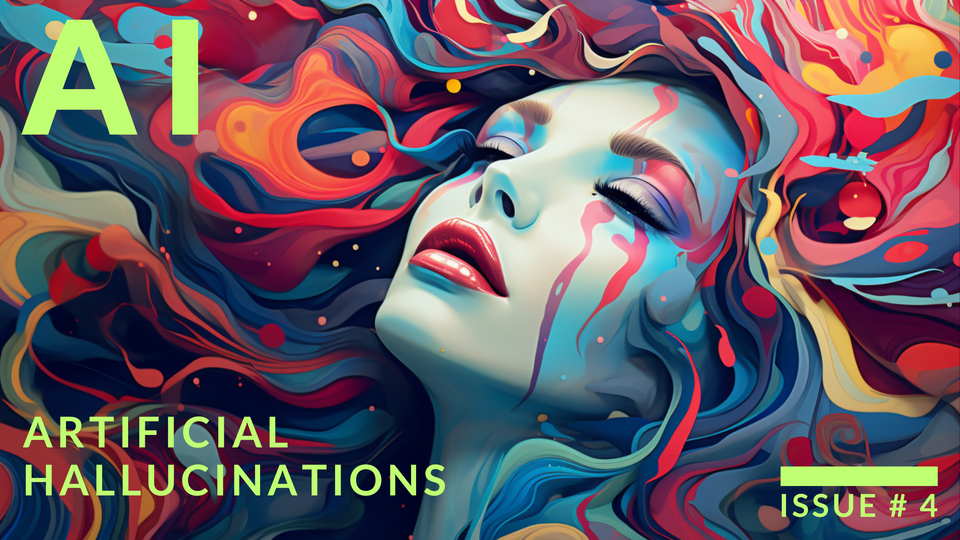
AI hallucinations, also known as hallucinatory responses, refer to outputs generated by artificial intelligence models that are not based on factual or coherent information. These outputs can be imaginative, nonsensical, or even contextually inappropriate, resembling the phenomenon of hallucinations experienced by humans in altered mental states. AI hallucinations occur due to various factors, including the way AI models are trained and the limitations in their understanding of context.
AI models, particularly those based on generative approaches like GPT-3, can sometimes generate content that appears to be plausible on the surface but lacks accurate information or logical coherence. This can happen for several reasons:
Lack of Contextual Understanding: AI models might not fully comprehend the nuances of a given context or topic, leading to responses that seem relevant but are actually inaccurate or fanciful.
Training on Diverse Data: AI models learn from a vast amount of text data, which can include misinformation, fictional content, and speculative discussions. This diverse training data might contribute to generating responses that are imaginative but not necessarily factual.
Creative Generative Process: Some AI models are designed to be creative and generate novel content. While this can lead to interesting outputs, it can also result in responses that deviate from reality.
Noise in Data: Noise in the training data, such as typographical errors, unusual phrasings, or outliers, can influence the AI's output and contribute to the generation of hallucinatory content.
Prompt Ambiguity: If the prompt provided to the AI is ambiguous or poorly constructed, the generated response might reflect a creative interpretation that strays from factual information.
AI hallucinations can range from harmless and amusing to potentially misleading or concerning. They highlight the challenge of ensuring that AI-generated content aligns with accurate and reliable information, especially in contexts where users might rely on AI for accurate insights.
To mitigate AI hallucinations, it's crucial to:
- Provide Clear and Contextual Prompts: Clear and specific prompts can help guide AI models toward generating relevant and accurate responses.
- Implement Contextual Checks: AI systems should be designed to assess the context and verify the accuracy of the generated content before presenting it to users.
- Post-Processing and Review: Implement human review processes to identify and filter out hallucinatory or inaccurate content.
- Continuously Improve Training Data: Enhance the training data by filtering out unreliable sources and ensuring a higher quality of information.
While AI hallucinations are a challenge, they also emphasize the ongoing need for research and development to refine AI models and ensure that their outputs are aligned with factual information and user expectations.
On the next article i will cover OpenAI Playground.
Thank you for visiting my blog!
Oscar Sosa